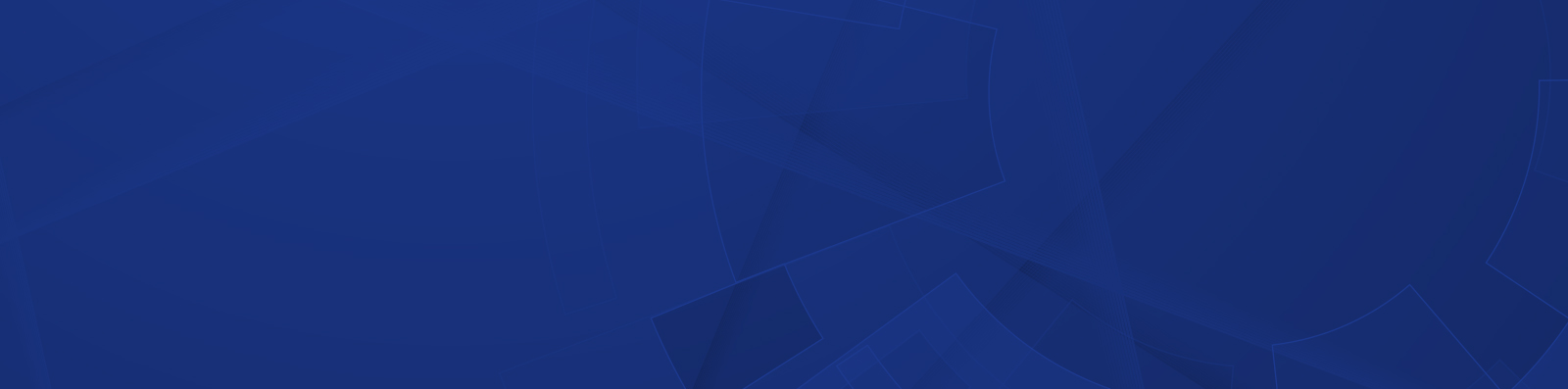
By Ryan Koonce
These days, customers find your product through a variety of marketing channels (ad platforms, partnerships, content, organic, etc.). It is important to understand how these channels work together in driving conversions. After all, the journey of the converted customer is the one we really care about, the one we want to promote and replicate. To understand this journey, we use multi-channel attribution modeling.
Let’s say the follow diagram represents your customer’s journey, how much credit does each channel deserve? Let’s explore several ways distribute the credit.
First click attribution gives 100% of the credit to the first touchpoint. In our example, display would get 100% of the credit.
First click attribution is useful for figuring out how customers original found your product, but doesn’t shed much light onto the conversion driving touchpoints.
First click attribution is akin to giving my first girlfriend 100% of the credit for me marrying my wife.
– Avinash Kaushik
Although I love this quote, it’s not accurate. First click attribution is actually akin to giving your friend that introduced you to your wife full credit for your marriage.
Last click attribution gives 100% of the credit to the last touchpoint. In our example, remarketing would get 100% of the credit.
Last click or last interaction is the classic model used in many reporting tools. It’s only good for figuring out which touchpoints are driving the actual conversions, it completely ignores the rest of the referral touchpoints.
Linear attribution is the most basic way of dividing a conversion. It divides the credit equally among each of the referring touch points.
This model is useful when analyzing a conversion event that has long sales cycles, where all the touch points are important in building a brand image.
The time decay model is the most advanced model we provide. It divides credit to each filter based on the number of days before the conversion.
The calculation we use for this is:
y = 2-x/7
where x is the number of days the referral happened prior to the conversion. The 7 in the equation is the half-life. A touchpoint 7 days before a different touchpoint, will receive half the credit.
For example, a user visits your site from a Google display ad, a remarketing ad and then finally a social channel, with the following timeline:
Based on the equation above, we would split the credit up for each channel accordingly:
DISPLAY | REMARKETING | SOCIAL |
---|---|---|
2-8/7 | 2-4/7 | 2-1/7 |
.453 | .673 | .906 |
22.29% | 33.13% | 44.58% |
The truth of the matter is, there’s no silver bullet for modeling attribution. Our team has found the best way to get an accurate understanding, is to compare our numbers for each of the models. This is easy to do using the model selector in Attribution. Give it a try with our demo data.
Navigating Through Walled Gardens in Commerce Most ecommerce platforms operate as walled gardens, holding large…
Your marketing efforts may be measured on a specific set of outcomes, such as more…
In today's fast-paced digital landscape, businesses are constantly seeking ways to gain deeper insights into…